Description
We introduce TNE, a generic framework to enhance the embeddings of nodes acquired by means of random walk-based approaches with topic-based information. Similar to the concept of topical word embeddings in Natural Language Processing, the proposed model first assigns each node to a latent community with the favor of various statistical graph models and community detection methods, and then learns the enhanced topic-aware representations. We evaluate our methodology in two downstream tasks: node classification and link prediction. The experimental results demonstrate that by incorporating node and community embeddings, we are able to outperform widely-known baseline NRL models.
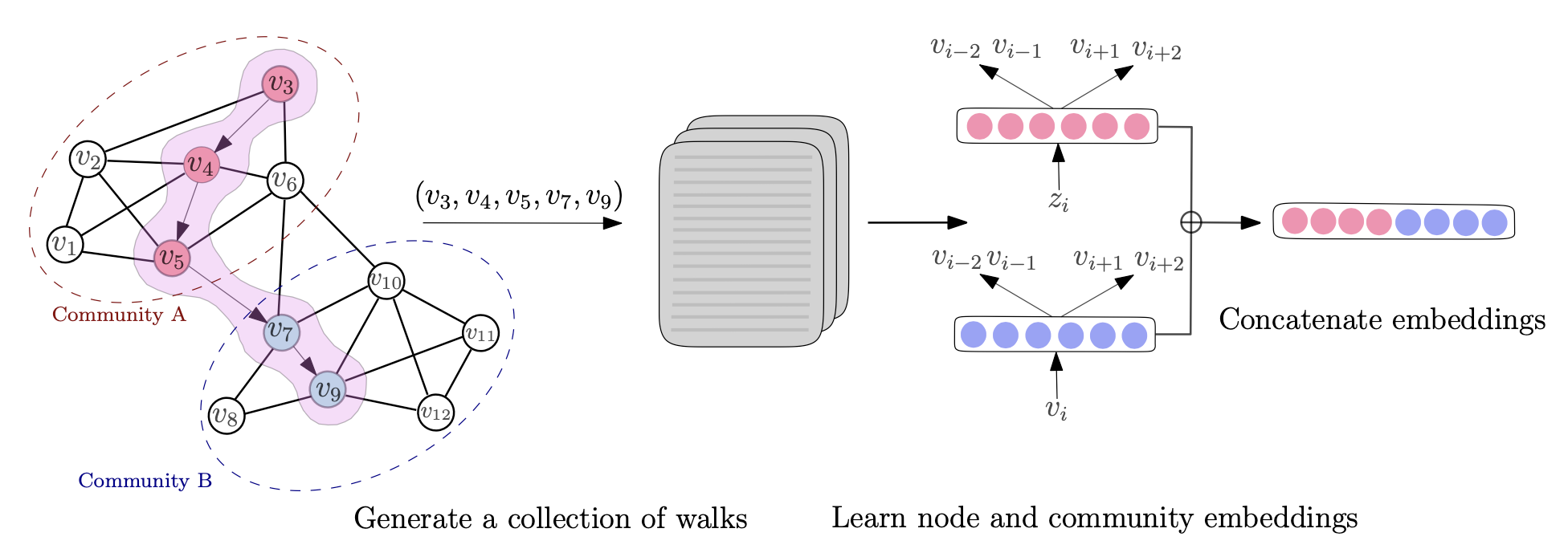
Code
The implementation of the TNE framework in Python can be reached at the Github repository.
Contributors
Abdulkadir Çelikkanat and Fragkiskos D. Malliaros
References
A. Celikkanat and F. D. Malliaros, TNE: A Latent Model for Representation Learning on Networks, NeurIPS Relational Representation Learning Workshop (NeurIPS-R2L), Montréal, Canada, 2018
A. Celikkanat and F. D. Malliaros, Topic-aware latent models for representation learning on networks, Pattern Recognition Letters, 2021